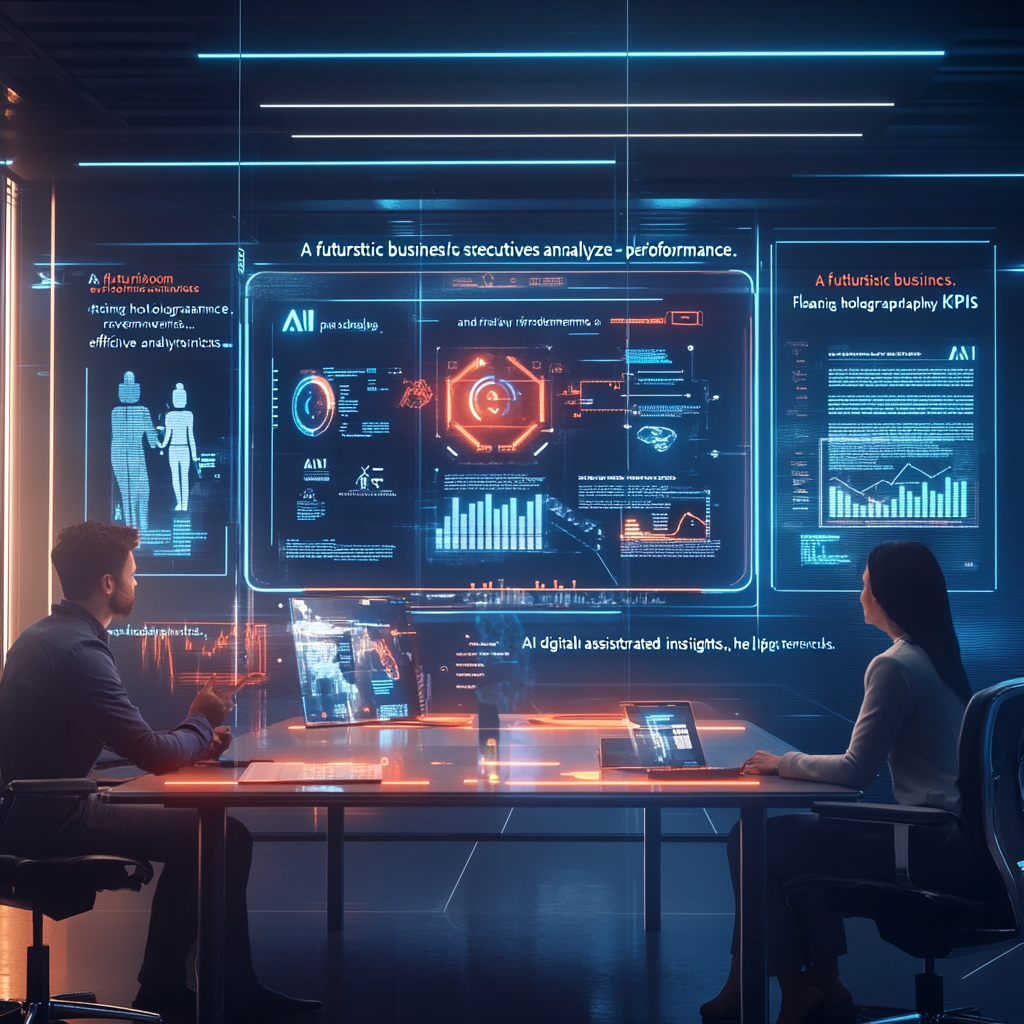
Measuring AI Impact: KPIs and Metrics That Matter
Introduction: AI is an Investment—Are You Measuring Its Success? AI [...]
Introduction: AI is an Investment—Are You Measuring Its Success?
AI adoption is accelerating across industries, but many businesses struggle to quantify its real impact.
Only 20% of companies have defined AI success metrics, leading to:
AI projects that fail to deliver measurable ROI.
Difficulty scaling AI initiatives beyond pilot phases.
Unclear AI adoption strategies due to lack of performance tracking.
AI is an investment—not an experiment. To ensure AI drives business value, companies must implement clear, data-driven KPIs that measure efficiency, ROI, model performance, and strategic impact.
In this guide, Blu outlines a structured AI performance measurement framework, helping businesses track AI’s success and optimize AI-driven decision-making.
The 4 Key AI Performance Metrics Every Business Must Track
1. Business ROI & Financial Impact Metrics
Key Question: Is AI improving revenue, cost savings, and profitability?
The Challenge: Many businesses invest in AI without defining clear financial KPIs, making it difficult to justify AI investments to stakeholders.
KPIs to Track:
- Revenue Growth from AI (e.g., AI-driven personalization increasing sales).
- Cost Reductions from AI Automation (e.g., process efficiency savings).
- Return on AI Investment (ROAI) (measuring AI costs vs. financial benefits).
Blu’s Approach: We help businesses define financial AI impact metrics and build AI-driven cost-benefit analysis frameworks.
2. AI Operational Efficiency & Productivity Metrics
Key Question: Is AI improving internal processes and employee productivity?
The Challenge: AI adoption often lacks measurable operational KPIs, making it unclear whether AI enhances efficiency or adds complexity.
KPIs to Track:
- Process Automation Rate (percentage of tasks automated by AI).
- Time Savings from AI (reduction in manual processing time).
- Error Reduction Rate (how AI improves accuracy in decision-making).
Blu’s Approach: We design AI performance dashboards that track automation rates, efficiency improvements, and productivity gains.
3. AI Model Performance & Accuracy Metrics
Key Question: How well are your AI models performing?
The Challenge: AI models require continuous monitoring to ensure accuracy, fairness, and reliability over time.
KPIs to Track:
- Model Accuracy & Precision (how well AI predictions align with actual outcomes).
- False Positives & False Negatives (measuring AI decision errors).
- Model Drift Rate (how AI performance changes over time).
Blu’s Approach: We implement AI model monitoring solutions to track accuracy, detect bias, and optimize performance over time.
4. AI Adoption & User Engagement Metrics
Key Question: Are employees and customers successfully using AI tools?
The Challenge: Many AI projects fail due to low user adoption rates and lack of employee engagement.
KPIs to Track:
- AI Adoption Rate (percentage of employees using AI-powered tools).
- User Satisfaction Scores (feedback from employees/customers on AI usability).
- Time to Value (TTV) (how long it takes AI to deliver measurable benefits).
Blu’s Approach: We conduct AI adoption assessments to ensure AI tools are integrated into daily workflows effectively.
A Step-By-Step Framework for Measuring AI Performance
Step 1: Define Business-Aligned AI Goals
✔ Align AI initiatives with financial, operational, and strategic goals.
✔ Ensure AI metrics reflect real business impact.
Step 2: Establish AI KPI Dashboards & Tracking Systems
✔ Implement real-time AI performance dashboards.
✔ Use AI-driven analytics tools to collect & analyze KPI data.
Step 3: Monitor AI Model Performance & Adapt Strategies
✔ Regularly audit AI accuracy, bias, and reliability metrics.
✔ Continuously optimize AI models to maintain high performance.
Step 4: Track AI Adoption & Workforce Engagement
✔ Gather feedback from employees & customers on AI usability.
✔ Improve AI training programs to increase adoption rates.
Step 5: Iterate & Optimize AI for Long-Term Success
✔ Adjust AI strategies based on performance insights & business needs.
✔ Scale AI adoption to new business areas based on proven impact.
Final Thoughts: AI Success Requires Clear Metrics
AI implementation without defined success metrics leads to wasted investments and unclear business impact. Companies that track AI performance effectively will:
Maximize AI ROI by aligning AI with measurable business outcomes.
Improve AI model accuracy & efficiency through continuous optimization.
Increase AI adoption by tracking and enhancing user engagement.
At Blu, we help enterprises implement custom AI performance measurement frameworks, ensuring AI delivers measurable business value.
Want to track your AI’s success? Let’s talk.
Share this article
Follow us
A quick overview of the topics covered in this article.
Latest articles
April 29, 2025
April 22, 2025
April 9, 2025