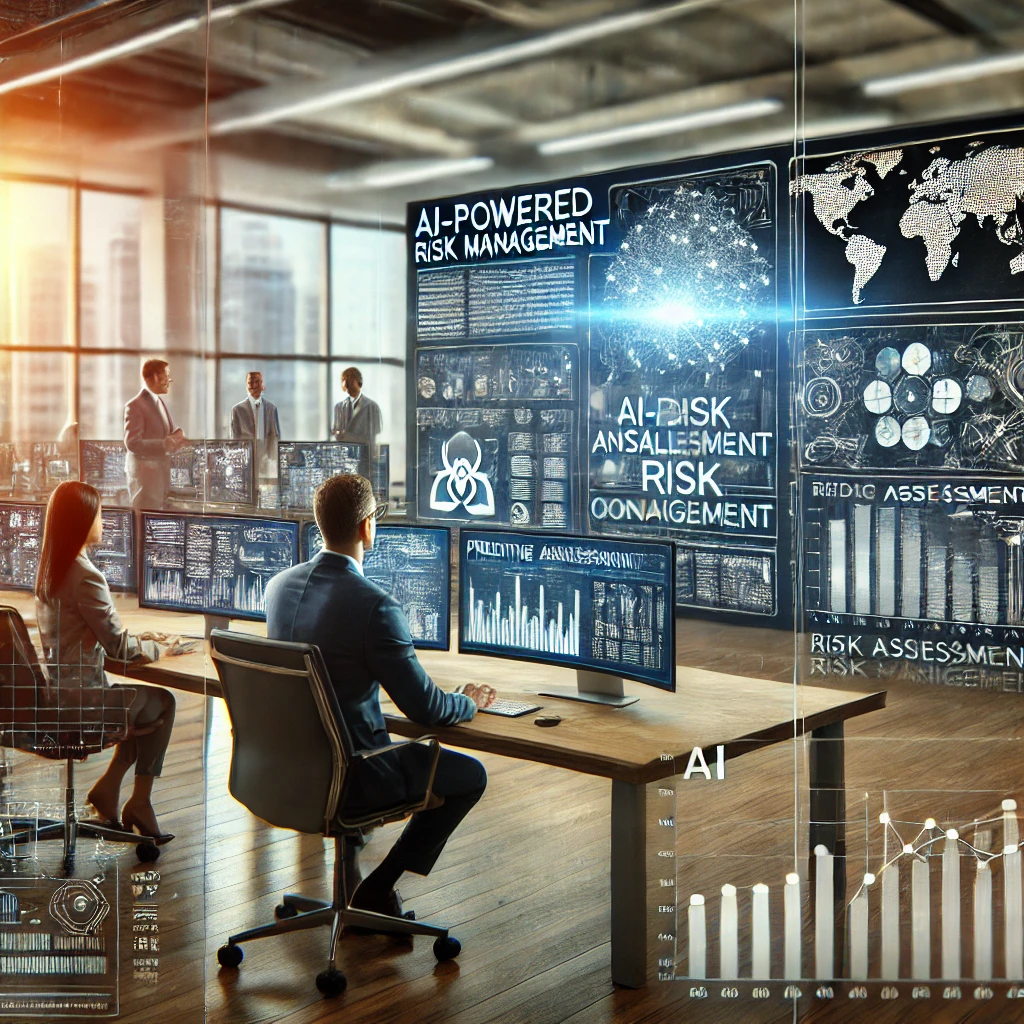
AI-Powered Risk Management: Anticipating and Mitigating Organizational Threats
In an increasingly complex and volatile business environment, effective risk [...]
In an increasingly complex and volatile business environment, effective risk management is more critical than ever. Traditional risk management strategies, while valuable, are often limited by their reliance on historical data and human intuition. With the advent of artificial intelligence (AI), organizations can now anticipate and mitigate risks more proactively and accurately. In this article, we explore how AI-powered risk management is transforming the way organizations identify, assess, and respond to potential threats.
The Evolution of Risk Management with AI
Traditional risk management approaches typically involve analyzing past events to predict future risks. While this method provides some insights, it can be limited by the availability and accuracy of historical data. AI, on the other hand, can process vast amounts of real-time data, identify emerging trends, and detect patterns that may not be apparent to human analysts. This allows organizations to anticipate risks before they fully materialize and to respond more swiftly and effectively.
Key Advantages of AI-Powered Risk Management
- Predictive Analytics: AI algorithms can analyze historical and real-time data to predict potential risks. By identifying patterns and correlations, AI can forecast future events with greater accuracy, enabling organizations to take preemptive action.
- Enhanced Detection: AI-powered systems can continuously monitor for signs of emerging risks, such as financial irregularities, cybersecurity threats, or supply chain disruptions. This real-time detection allows organizations to address issues before they escalate.
- Scenario Simulation: AI can simulate various risk scenarios, helping organizations understand the potential impact of different threats and plan accordingly. These simulations can be used to develop contingency plans, allocate resources, and prioritize risk mitigation efforts.
- Automated Decision-Making: AI can assist in the decision-making process by providing data-driven recommendations on how to manage identified risks. This can include suggesting specific actions, optimizing risk response strategies, and automating routine risk management tasks.
Implementing AI-Powered Risk Management
- Integrate AI with Existing Risk Management Systems: Start by integrating AI into your current risk management framework. This could involve adding AI-driven analytics to existing dashboards, using AI to enhance data collection, or incorporating AI-powered tools into risk assessment processes.
- Leverage Real-Time Data: AI-powered risk management systems thrive on real-time data. Ensure that your organization has access to up-to-date information across all relevant areas, such as financial data, market trends, customer behavior, and regulatory changes. The more comprehensive the data, the more effective the AI system will be at identifying risks.
- Develop AI-Driven Risk Models: Work with data scientists and risk management professionals to develop AI-driven risk models tailored to your organization’s specific needs. These models should be designed to identify the types of risks most relevant to your industry, such as financial risks, operational risks, or reputational risks.
- Train and Support Your Team: Ensure that your risk management team is trained to work with AI-powered tools. This includes understanding how to interpret AI-generated insights, how to act on AI recommendations, and how to continuously monitor and refine AI systems to ensure they remain effective.
Case Studies: AI-Powered Risk Management in Action
- Financial Services: A major bank implemented AI-powered risk management tools to detect fraudulent transactions in real-time. The AI system identified patterns of suspicious activity that human analysts had missed, resulting in a significant reduction in fraud losses.
- Manufacturing: A global manufacturing firm used AI to monitor its supply chain for potential disruptions. The AI system identified early warning signs of supplier issues, allowing the company to take proactive steps to mitigate the impact on production.
Challenges and Considerations
- Data Quality: The effectiveness of AI-powered risk management depends on the quality of the data used. Organizations must ensure that their data is accurate, complete, and free from bias to avoid skewed or misleading results.
- Ethical Considerations: AI systems must be designed and deployed in ways that respect ethical principles, such as fairness, transparency, and accountability. Organizations should establish guidelines to ensure that AI-driven decisions align with their values and do not disproportionately impact certain groups.
- Integration with Human Judgment: While AI can enhance risk management, it should not replace human judgment entirely. Organizations should use AI as a tool to support decision-making, with final decisions being made by experienced professionals who can consider the broader context.
AI-powered risk management offers organizations a powerful new way to anticipate and mitigate potential threats. By leveraging AI’s predictive capabilities, real-time detection, and scenario simulation, businesses can stay ahead of emerging risks and make more informed decisions. At Blu, we’re committed to helping organizations harness the power of AI to enhance their risk management strategies and protect their assets. Contact us today to learn how AI-powered risk management can benefit your organization.
Share this article
Follow us
A quick overview of the topics covered in this article.
Latest articles
April 29, 2025
April 22, 2025
April 9, 2025